i2G Machine Learning Toolkit has been successfully utilized to tackle various G&G challenges from data correction, missing logs prediction to rock type classification and so on. It offers a wide range of regression and classification methods from linear/non-linear regression to more complex algorithms of Artificial Neural Network and Random Forest. Powerful i2G cloud-based machine learning enables its users to easily build petrophysical machine learning model based on limited data availability which can be later scaled up when new data comes in. The i2G machine learning projects have full access to data from multiple working projects for different fields/blocks, creating a unique way to share “knowledge” from the data but the data itself.
Below is a successful case study of using i2G random forest regression to predict missing porosity logs of Neutron and Density from Gamma Ray and Resistivity curves. There are 45 wells in the database and only 11 wells with full conventional logs available because the studied interval was overlooked at the early exploration stage. Other remaining 34 wells have only Gammy Ray and Resistivity which makes it nearly impossible to accurately calculate porosity and hence water saturation and net res/pay. The machine learning models were trained and validated using data from wells with full conventional logs and then making Neutron and Density predictions for the remaining wells. Eventually, with porosity logs now available our client can apply their conventional petrophysical workflow to calculate reservoir properties in 1D and populate them into a 3D reservoir model – a critical component in the development plan for this interval.
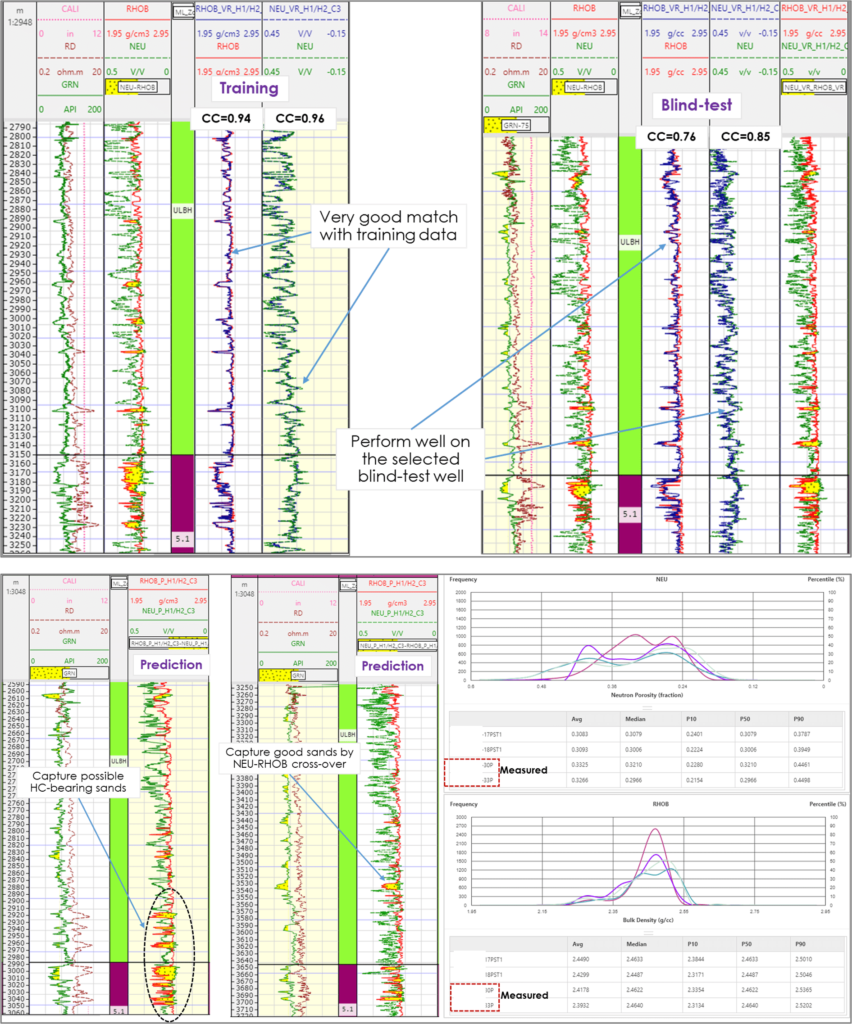
The i2G Random Forest is an ensemble technique capable of performing both regression and classification tasks with the use of multiple decision trees and a technique called Bootstrap. Random Forest method overcomes the nature of being overfitting to the training set when using a single decision tree. Well logs data that were fed into the process of training, validation and prediction required data harmonization which was basically a step to establish a database of properly corrected and normalized logs. The workflow was also assisted by i2G Python Scripting for batch processing due to working with a relatively large number of wells.
We are currently expanding this study to identify and characterize missed pay intervals, focusing on thin bed and low resistivity layers.